Documentation
Baseten is a platform for deploying and serving AI models performantly, scalably, and cost-efficiently.
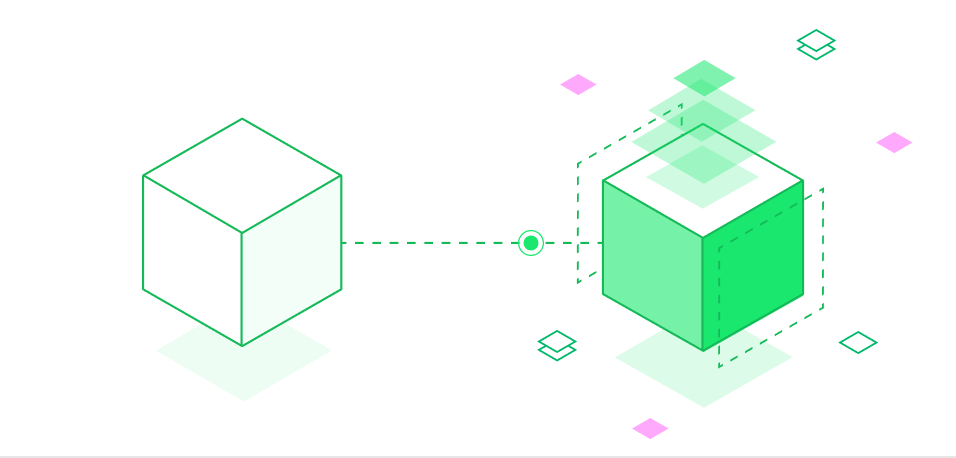
Quick start
Choose from common AI/ML usecases and modalities to get started on Baseten quickly.
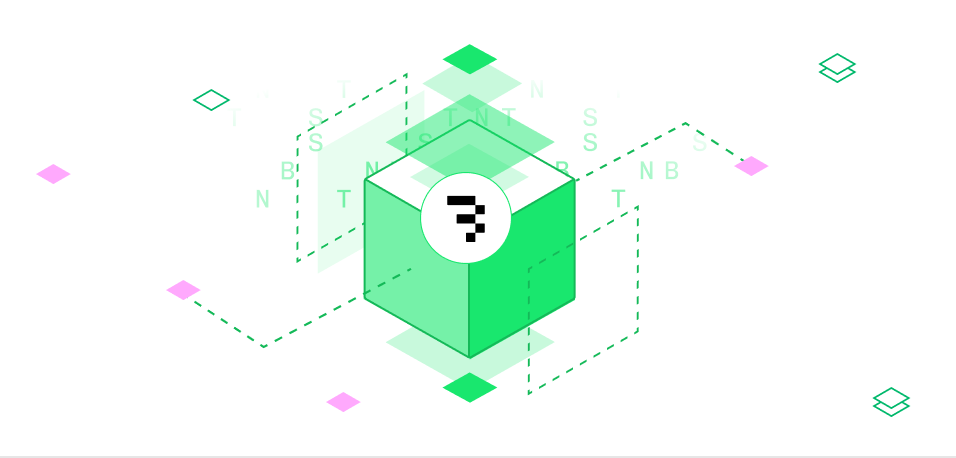
How Baseten works
Baseten makes it easy to deploy, serve, and scale AI models so you can focus on building, not infrastructure.
Baseten is an infrastructure platform for AI/ML models that lets you:
-
Package any model for production: Define dependencies, hardware, and custom code without needing to learn Docker. Build with your preferred frameworks (e.g. PyTorch, transformers, diffusers), inference engines (e.g. TensorRT-LLM, SGLang, vLLM), and serving tools (e.g. Triton) as well as any package installable via
pip
orapt
. -
Build complex AI systems: Orchestrate multi-step workflows with Chains, combining models, business logic, and external APIs.
-
Deploy with confidence: Autoscale models, manage environments, and roll out updates with zero-downtime deployments.
-
Run high-performance inference: Serve synchronous, asynchronous, and streaming predictions with low-latency execution controls.
-
Monitor and optimize in production: Monitor performance, debug failures, and export metrics with built-in observability tooling.
Resources