Build with Baseten
Baseten is a platform for deploying and serving AI models performantly, scalably, and cost-efficiently.
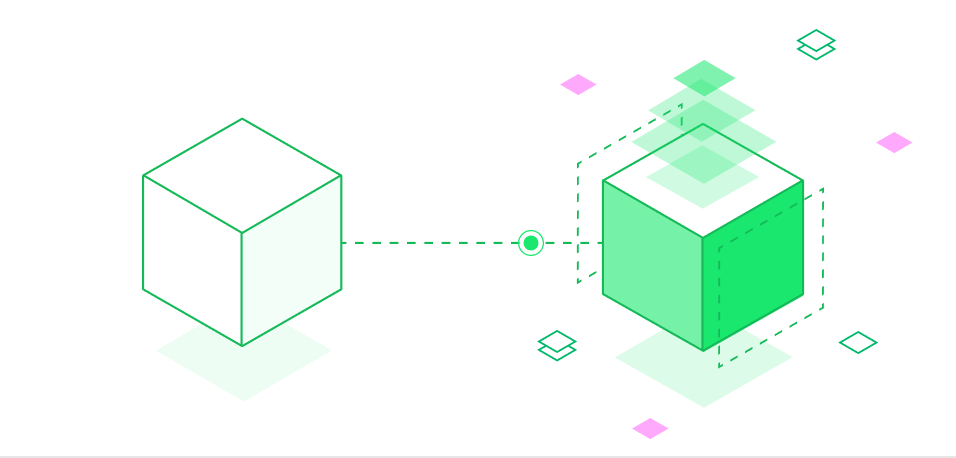
Quick start
Choose from common AI/ML usecases and modalities to get started on Baseten quickly.
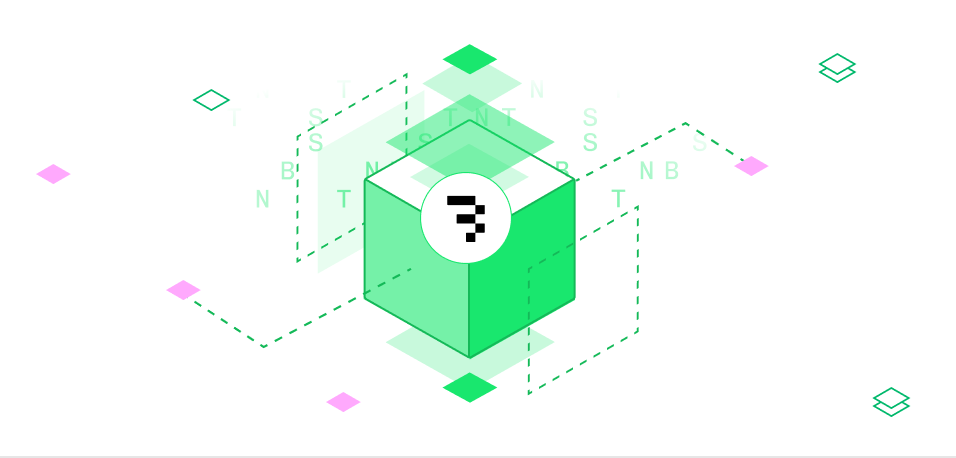
How Baseten works
Baseten makes it easy to deploy, serve, and scale AI models so you can focus on building, not infrastructure.
Baseten is an inference and training platform that lets you:
Deploy dedicated models with full control
-
Package any model for production: Define dependencies, hardware, and custom code without needing to learn Docker. Build with your preferred frameworks (e.g. PyTorch, transformers, diffusers), inference engines (e.g. TensorRT-LLM, SGLang, vLLM), and serving tools (e.g. Triton) as well as any package installable via
pip
orapt
. -
Build complex AI systems: Orchestrate multi-step workflows with Chains, combining models, business logic, and external APIs.
-
Deploy with confidence: Autoscale models, manage environments, and roll out updates with zero-downtime deployments.
-
Run high-performance inference: Serve synchronous, asynchronous, and streaming predictions with low-latency execution controls.
-
Monitor and optimize in production: Monitor performance, debug issues, and export metrics with built-in observability tooling.
Start fast with model APIs
- Try model APIs: Model APIs provide a fast path to production with reliable, high-performance inference. Use OpenAI-compatible endpoints to integrate models like Llama, DeepSeek, and Qwen, with built-in support for structured outputs and tool calling.
Pre-train and fine-tune models
-
Run training jobs on scalable infrastructure: Launch containerized training jobs with configurable environments, compute (CPU/GPU), and resource scaling. Supports any training framework via a framework-agnostic API.
-
Manage artifacts and streamline workflows: Track experiments, organize training runs, and handle large artifacts like checkpoints and logs. Seamlessly transition from training to deployment within the Baseten ecosystem.
Resources